Rupa Kurinchi-Vendhan
Computer Vision Researcher
for Sustainability and Conservation
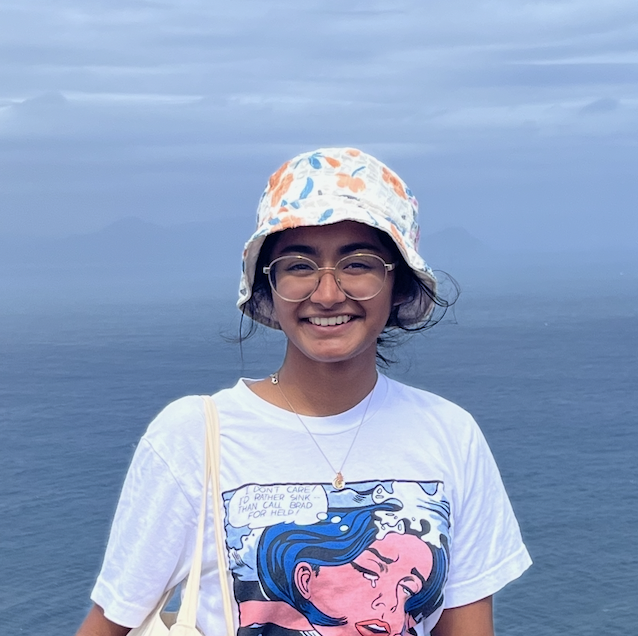
I am a first-year PhD student at MIT CSAIL, advised by Professor Sara Beery and supported by the MIT Tina Chan Fellowship. I am interested in the intersection of AI and ecology, furthering the domain of computer vision by leveraging the constraints of the natural world. Specifically, my research focuses on multimodal systems, learning from remote sensing images to abstract soundscapes--to address climate issues such as conservation and biodiversity monitoring. I am broadly interested in working at the intersection between research and policy, working closely with
stakeholders to design and deploy real-world ecological tools. Previously, I received my bachelor's degree at the California Institute of Technology in Computer Science. Throughout my undergraduate career, I was advised by Professor Pietro Perona (Caltech), Professor Steven Low (Caltech), and Professor Dava Newman (MIT) on various remote sensing-based computer vision projects for species distribution modelling and solar and wind mapping. Aside from academic research, I have completed internships with NASA Langley, Apple, and Coral Gardeners.
Rupa Kurinchi-Vendhan, Drew Gray, Elijah Cole, and Pietro Perona
Developed a novel transformer-based neural network capable of multi-label benthic classification, taking processed drone imagery as input and identifying pixels as coral cover, rocks, rubble, sand, algae, etc. This model will be used to inform restoration efforts by providing actionable, specific evidence of where corals should be planted (in areas of higher relative live coral cover).
Edward Cronin, Ashley Fernando, Jarrett James, Rupa Kurinchi-Vendhan
Through NASA's DEVELOP National Program, we worked with the Langley Research Center (LaRC) and partnered with Washington DC's Department of Energy and Environment (DOEE) to create solar potential maps to inform solar panel installation decisions for the District.
Rupa Kurinchi-Vendhan, Björn Lütjens, Ritwik Gupta, Lucien Werner, Dava Newman, Steven Low
An accepted paper at the NeurIPS CCAI Tackling Climate Change with Machine Learning 2021 Workshop. We modified existing deep learning-based super-resolution models, and applied them to satellite data to increase the resolution of wind speeds and solar irradiance fields for informing short-term, local energy planning. We published machine learning-ready wind and solar datasets.
Rupa Kurinchi-Vendhan, Drew Gray, Elijah Cole, Pietro Perona
arXiv pre-print arXiv:2311.13661 | 2023
Rupa Kurinchi-Vendhan, Björn Lütjens, Ritwik Gupta, Lucien Werner, Dava Newman
NeurIPS CCAI Tackling Climate Change with Machine Learning Workshop | 2021
Edward Cronin*, Ashley Fernando*, Jared James*, Rupa Kurinchi-Vendhan*
NASA Technical Reports | 2021